Robotics and automation are hardly new in food production, says Chef Robotics founder Rajat Bhageria. “The issue with traditional automation is that it’s not flexible.”
Historically, observes Bhageria, who has been keeping a low profile since founding his AI-powered robotics startup in 2019, robots have been designed to automate specific tasks in ‘low mix’ production lines optimized for mass production of a single product. But they do not suit ‘high mix’ environments where companies are handling hundreds of SKUs or producing customized meals.
In these more complex environments, or in situations where products are too delicate to be handled by traditional dispensers, he says, “traditional automation simply doesn’t work” and companies still rely heavily on manual labor.
San Francisco-based Chef Robotics has found its sweet spot with these companies, which have a certain level of complexity, but a meaningful level of throughput, where it can deploy robotic arms armed with proprietary utensils trained to dispense accurate portions of food into trays through rapid ‘on-the-job’ learning, facilitated by AI.
Sensing includes a few depth cameras, as well as a weight-sensing platform for the food container to ensure consistent amounts of food are picked, says Bhageria, a master’s graduate of Penn’s Robotics and Machine Learning Lab who started his first company in high school and launched an early-stage venture capital company called Prototype Capital in 2018 that has invested in several robotics startups.
After experiments with leading brands including Amy’s Kitchen, Chef Bombay, and Sunbasket, Chef’ Robotics’ technology is now being rolled out across North America, says Bhageria, who operates a Robotics as a Service (RaaS) model.
AgFunderNews caught up with Bhageria to talk imitation learning, shredded chicken, and the future of robots in food.
AFN: How is Chef Robotics using AI, and what data are you training it on?
RB: Our systems are based on advances in diffusion models and deep learning so our robotic arms are adaptable enough to pick and plate almost any ingredient.
But to have highly capable AI, you need training data. In purely cloud AIs like large language models, you can get that off the internet, while in self-driving cars, you can use simulations to generate data. But in the food industry, there’s no off-the-shelf training data and there are no physics-based simulation engines because food can be deformable, sticky, wet and inconsistent.
To generate useful training data, you need to deploy robots in actual production environments, which means we’re facing something of a chicken and egg situation [when approaching a new client].
So what we’ve been doing is initially deploying robots where we can partially automate an operation and thus still add value without requiring 100% autonomy from the get-go, but the more data we pick up from our deployments, the more our AI models improve.
Ultimately, as the AI becomes more and more autonomous and can handle more ingredients, we can then move from applications in industrial food production to every commercial kitchen in the country.
In other words, once the AI learns how to manipulate shredded chicken in a large industrial kitchen, it can leverage the same model to manipulate a similar shredded chicken in a commercial kitchen. Our training data has grown exponentially since the first deployment in 2022, so we’re becoming more flexible and able to handle a higher number of edge cases.
Both the dexterity and training data allow robots enabled by [proprietary software platform] ChefOS to have high precision inference and manipulate the ingredient while being consistent, not damaging it, not spilling it, and also being able to manipulate various portion sizes. Chef has now manipulated hundreds of ingredients in production.
AFN: Is Chef’s expertise more in AI and software than hardware?
RB: We believe that the winners in this space are going to be the ones with the most production training data. The hardware is mostly off the shelf except for the novel designs of the utensils, which are an important part of our IP.
But while the hardware is off the shelf, the systems integration is actually not trivial if you want to make these systems useful for the customer.
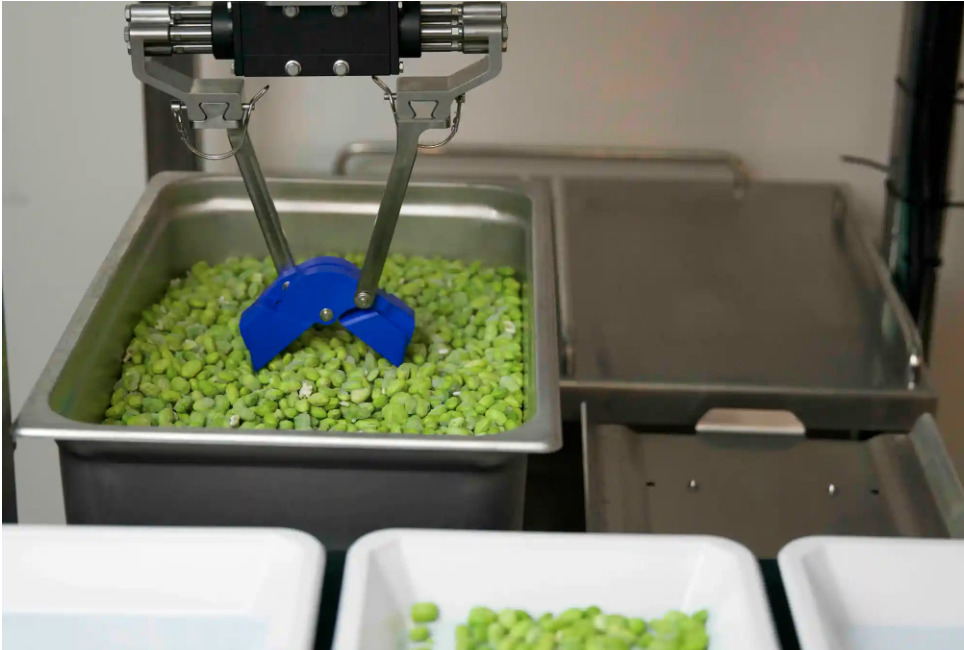
AFN: How is your ChefOS system collecting data?
RB: We have a lot of different sensors that allow us to understand things like how much pressure and force to apply to a given material as we pick it. Each robot has a different interface where you can attach different utensils and cameras pointing at the ingredient [being picked up from a tub/container] and the conveyor [containing trays in which food is deposited].
You basically slide the module onto the conveyor which is on casters, you plug in power and compressed air, and then on the touchscreen, the user inputs the meal that’s being run on the line, attaches the relevant utensils, and the robot gets to work.
AFN: How widely deployed is your technology and what’s the ROI for customers?
RB: Over the past two years, Chef has done 20 million servings in production, has robots deployed in six cities in the US and Canada, and has learned how to manipulate hundreds of ingredients.
When it comes to ROI, the biggest benefit to customers is making more revenue. If you have 10 lines but you can only run seven of them because you don’t have enough people, or someone didn’t show up for work, you’re missing out on revenue.
The second benefit we think a lot about is that average throughput goes up with robots. They don’t get tired, they are very consistent, they don’t need breaks, and they always show up for work.
Number three, they help reduce giveaway and increase yield. Humans always tend to over-deposit, put in a little bit too much, whereas robots are very consistent.
And finally of course, there’s the labor savings itself, and the fact that you can free up humans to do other tasks.
As an example, we helped Chef Bombay [a contract manufacturer of meals for major retailers in the US and Canada] reduce standard deviation by 30%, reduce food giveaway/wastage by 88%, increase labor productivity by 33%, and increase throughput by 9%.
At Amy’s Kitchen [which makes a wide large selection of ready meals], we improved product consistency by 12%, reduced food giveaway by 4%, and increased labor productivity by 17%. At Sunbasket—which is known as a B2C a meal kit company, they actually also contract manufacture meals for other brands and we have robots with them on the east and west coast. [Using Chef robots] they required 10% fewer people to run a line and saw a 17% increase in throughput.
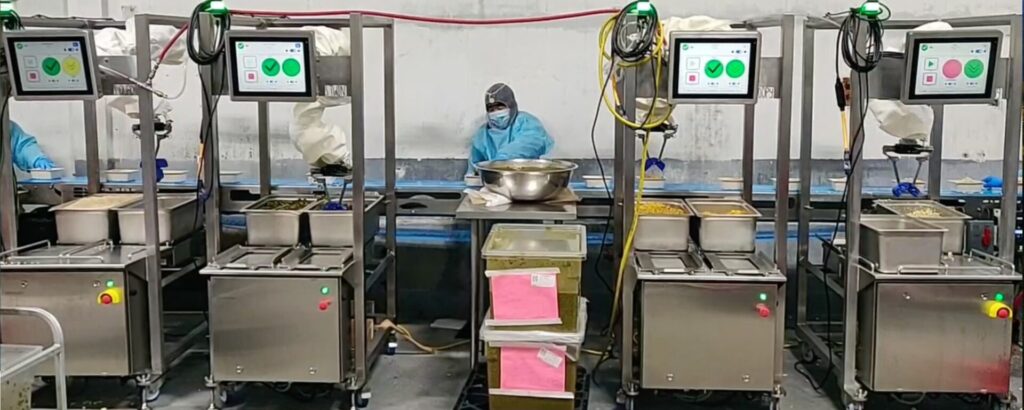
AFN: How have you funded the business?
RB: We’ve raised $18.2 million in equity and we raised another $4.25 million in equipment financing and debt. In terms of the fundraising environment, obviously the market is not great. But at the same time, I think what investors appreciate about us is we have been very practical.
A lot of robotics companies have made big grandiose promises, but they haven’t really shipped any robots. We’ve been able to get some big names in the food industry and we have happy customers.
In the restaurant space, there are a ton of robotics players but in many cases they build a cool robot, but didn’t really solve a problem, or they try to solve the wrong problem. So say you’re cooking burgers. That’s something a chef can do pretty well at scale and the robots aren’t perfect, so you still need someone to watch over them.
AFN: How has your vision for Chef Robotics evolved since you started out in 2019?
RB: It was clear there were labor issues in the food industry, but the question was, what’s the main task we should focus on? Should we focus on restaurants, ghost kitchens, food manufacturing? What we learned is that if we wanted to do something like a ghost kitchen or a fast casual restaurant, we don’t have the training data to be able to help right away.
Think about a salad chain like Sweetgreen or Mixt. They don’t have enough volume, they’re not making tens of thousands of meals, it’s more like hundreds of meals a day, and if you want to be useful you really need to be able to handle all of the tasks and ingredients, or you still need someone on the assembly line. To add value you’d need to fully automate and if you can only do 20% of the tasks it’s actually not that useful.
So then we looked at high-mix manufacturing, which is a huge category, so for example, if you make fresh or frozen prepared meals, patient trays for hospitals or airplanes or schools. Those companies change menus every week or month and they have hundreds of SKUs, so they have to be flexible, so on each line you have humans that are scooping food out of big tubs into individual trays as they move down the line.
We went to a bunch of these plants and it was a case of holy crap, this is perfect. This is where we need to start. So we have a roll up module with the same footprint as a human; it just slides onto the line and we can bootstrap that training data set in our lab for say five ingredients, and make the robot just good enough to ship something to production.
And then as soon as we get something in production and we get a real-world training data about how you manipulate food, it gets better and better, so over time, we can have this system that can help automate these high mix production facilities.
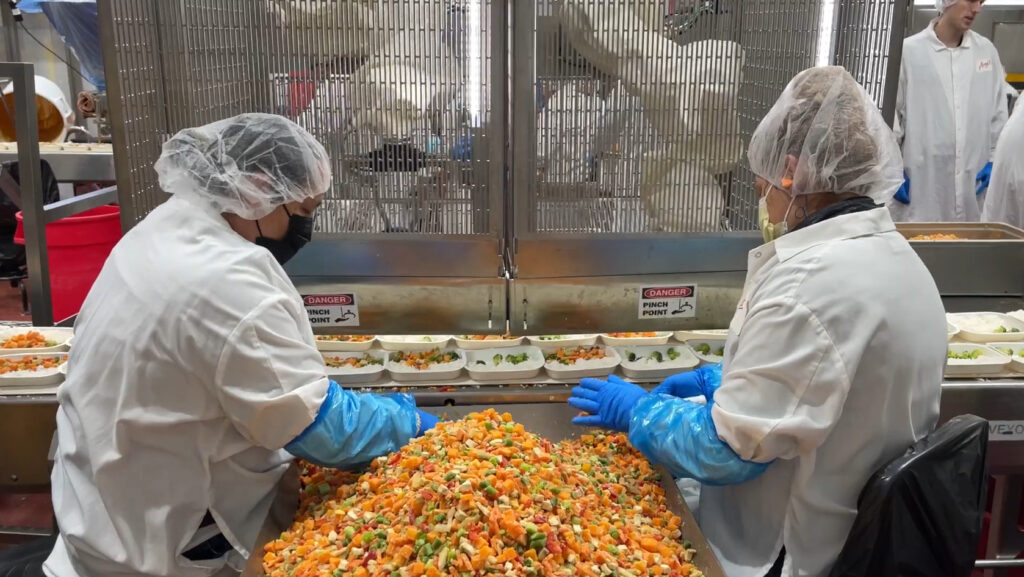
AFN: What are the biggest barriers to uptake? Why do potential clients say ‘No’?
RB: First, this is new technology, and anytime you have a new product you have to educate the market a little bit, but for us, having customers speak for us has really helped. We actually don’t need tens of thousands of customers; because of our business model of working with fairly big customers with multiple plants, just 20 customers could get us to a really large volume.
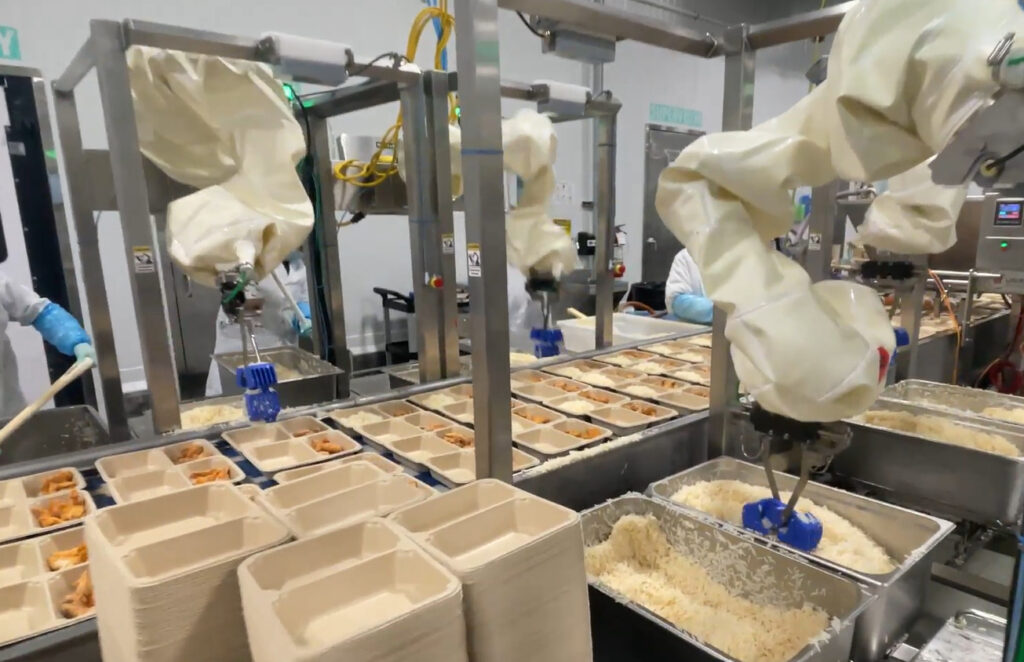
AFN: What excites you about the future of robotics in food?
RB: If you look at something like a car plant where you have a robot arm, it’s doing high volume and low mix, and that’s existed for 50 years, I think what’s happening now is advances in AI are allowing us to do high volume and high mix.
You want to be able to rapidly change over a line and go from one SKU to another, from mashed potatoes to cheese to chicken; that’s what we’re doing today. But if you look at what we’re ultimately aiming for with robotics, it’s high mix, low-volume [restaurants], and I think that’s where the industry is going. But it will take time.
There’s a lot of new advancements in AI which will accelerate this, such as learning by demonstration and imitation learning. Historically, companies would make a robot policy for a given ingredient, say, so for diced chicken you’d have an engineer writing a policy about how you manipulate that chicken.
Now, what’s happening with learning from demonstration is you can basically have a human demonstrate a scooping motion using one robot, and the second robot will just mimic it. You can do 30 to 40 demonstrations and use that to train a diffusion policy and get this very articulate, very dexterous scooping motion.
And by the way, the training time it takes to generate that policy might be a couple hours, rather than a couple of weeks.
Sponsored
Sponsored post: The innovator’s dilemma: why agbioscience innovation must focus on the farmer first